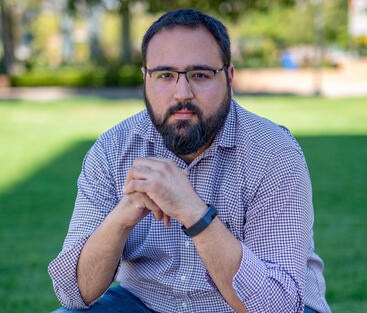
UCR Professor Vagelis Papalexakis has been named a winner of the Donald G. Fink Overview Paper Award presented by the Signal Processing Society of the Institute of Electrical and Electronics Engineers, or IEEE.
The award honors the authors of a journal article that has had substantial impact over several years on a subject related to the Signal Processing Society's technical scope.
Papalexakis was recognized for his work as a co-author on the paper “Tensor Decomposition for Signal Processing and Machine Learning,” published in IEEE Transactions on Signal Processing in 2017. Other researchers have since cited the paper 1,280 times, according to Google Scholar.
“I’ve always appreciated overview papers because they provide an entryway to the research area they cover, but never in my wildest dreams would I have predicted how well-received and impactful this overview paper would be to signal processing and machine learning researchers,” said Papalexakis, an associate professor of computer science and engineering in the Marlan and Rosemary Bourns College of Engineering.
It was the second IEEE award Papalexakis received this academic year.
He was earlier named the winner of the 2022 Tao Li Award presented this month at the International Conference on Data Mining in Orlando, Fla. This award goes to scholars in the field of data mining and machine learning who are either in a doctoral program or received a doctoral degree less than 10 years ago.
His research focuses on tensor (arrays indexed by three or more indices) methods for machine learning and data science.
Tensor methods, more specifically tensor decompositions, have been around for many decades, used first in psychometrics and chemometrics applications, and eventually finding their way to signal processing, machine learning, and data science.
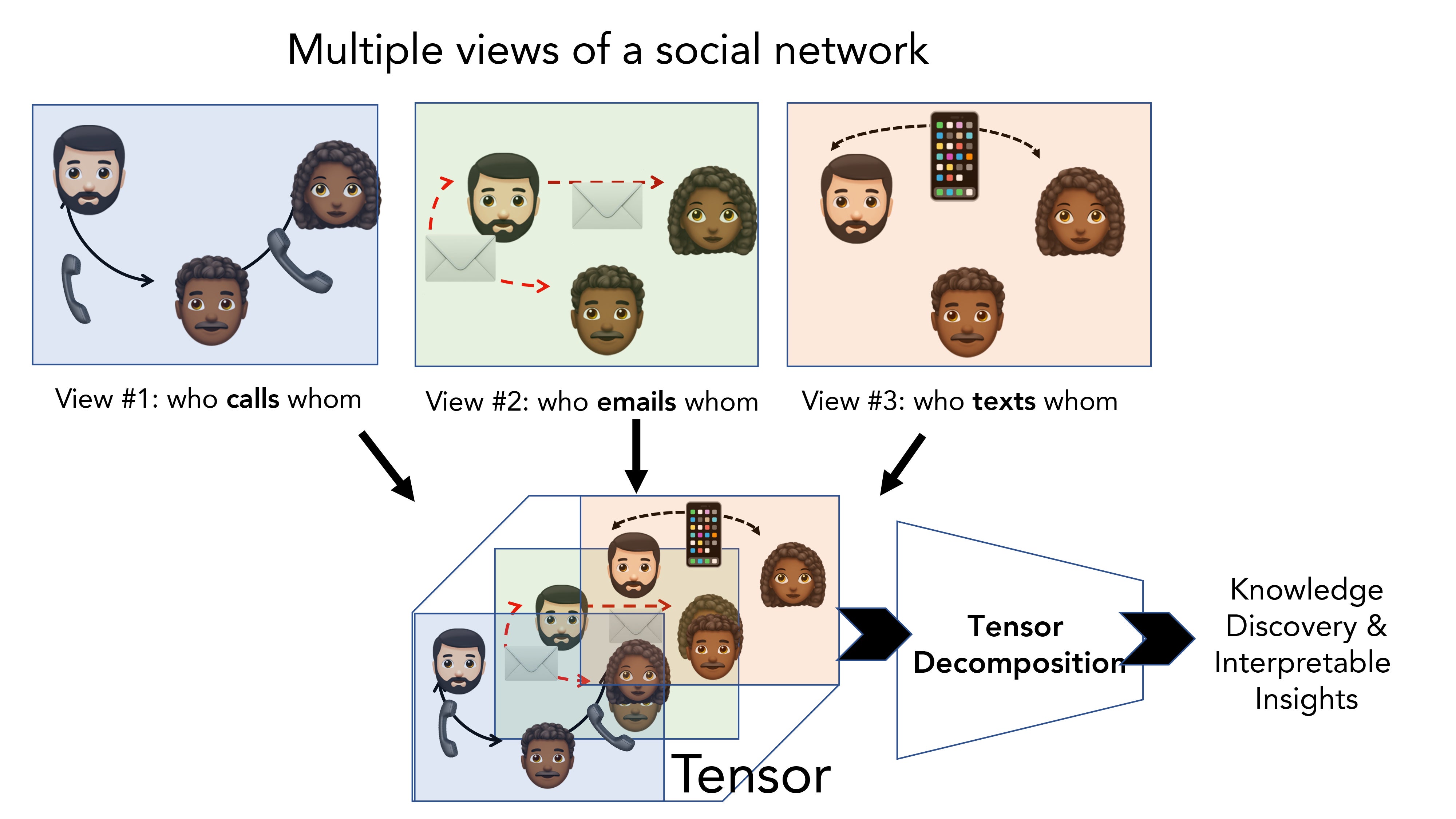
Papalexakis said he is fascinated by the versatility of those methods, which repeatedly have been shown to be transformative in different disciplines. Tensor methods are simultaneously very powerful, being able to model and extract knowledge from heterogeneous and diverse types of datasets, while at the same time lending themselves to simple interpretations of their results. As a result, tensor methods have had a wide array of applications, ranging from social network analysis to brain data analysis.
A major focus of Papalexakis’s research is applying tensor methods to misinformation detection, gravitational wave detection, explainable artificial intelligence, neuroscience, sports analytics and cybersecurity.
Papalexakis is a native of Athens, Greece, he joined UCR in 2016 after receiving his doctoral degree from Carnegie Mellon University in Pittsburgh.